Spectrometric technology can assess many critical characteristics about products, but it has limits. It can be challenging to determine when the line has been crossed
Within the technology category of analyzers, spectrometers provide a broad range of analytical capabilities and are available in an extensive range of designs from numerous suppliers. Spectroscopy has two main categories: atomic and molecular. Both examine the interaction between electromagnetic radiation and the sample being analyzed.
Atomic spectroscopy looks for specific elements that are present in samples — without regard to their chemical form. For example, refiners must determine and certify the sulfur content in diesel fuel. Though sulfur can be a component in dozens of different compounds that are present in the fuel, atomic spectroscopy selectively excites and detects all sulfur atoms — regardless of how they are bonded in larger molecules. This aggregated value satisfies the regulators’ objective to measure the overall sulfur content of the fuel.
By contrast, molecular spectroscopy examines the chemical bonds that are present in compounds. Instead of exciting specific atoms, this technology elicits telltale signals from the bonds between atoms. For example, methane gives distinct signals because of the characteristic way the bonds between its carbon and four hydrogen atoms respond to electromagnetic radiation.
This discussion focuses on molecular spectroscopy. Quantitative molecular spectroscopy is used in a wide variety of applications in many industries. Most relate to chemical quality assessments, such as examining a product to ascertain the desired component proportions, or the presence or absence of compounds that are considered to be contaminants. Consider a farmer bringing his or her grain harvest to the local grain elevator. The buyer can take a sample of the corn or wheat and use a near-infrared (NIR) spectrometer to check for critical attributes, such as moisture and food value in the form of oil and protein. The final price will likely be influenced by these quality measures, and the analysis can be done in a matter of minutes.
Another example could be a pharmaceutical producer trying to determine the moisture level in granules being processed in a dryer prior to tablet production. The technician can try to calculate it by measuring the humidity of the air leaving the dryer, but the NIR spectrometer provides a direct measurement that is automated, rapid and precise. Meanwhile, the device can be installed to perform the measurement online and provide measurements in realtime, allowing the process to stop immediately once the desired level has been reached.
These are relatively simple processes. Moisture is very easy to measure. Grain is a bit more complex, but a kernel of corn will have a short list of key attributes. Although there may be a variety of similar but technically different oils, they still tend to show up together, and an aggregate reading is valid for the application. When the list of possible chemical components grows, so does the difficulty of making accurate measurements.
Far more difficult is the application of molecular spectroscopy to assess the characteristics of gasoline, which is one of the most chemically complex products. Gasoline is not a single compound, but is a mixture of hundreds or thousands of different hydrocarbon molecules. Trying to measure various characteristics — such as octane rating — is not easy. This article examines the use of spectroscopy for this situation.
Characteristics and spectra
Chemical producers are always looking for better ways to measure some property of interest in a product. This can take countless forms. Picture a moonshiner loading a new batch of fermented mash into a still. He wants to know how much ethanol is in the mash, so he watches the temperature closely as it heats up. Why? Because he knows there is a direct and distinct relationship between the boiling point of the mixture and the proportion of ethanol to water. The composition is expressed by the boiling point.
A professional distiller could use a spectrometer instead of monitoring temperature, because the spectrum it generates will have features that correlate clearly and directly with component volumes. Similarly, an engineer may desire to control a chemical process on the basis of a known dependence of product quality or performance on some specific molecular property. Often, online molecular spectroscopy can be implemented to measure that property.
A good example is a production unit making polyols for use in polyurethane foams or sealants. The hydroxyl value must be controlled to tight specifications to ensure the proper NCO-to-OH ratio in reactions with isocyanate. The spectrometric analysis of hydroxyl number in a minute is possible because of a direct correlation with the NIR signal for the hydroxyl group in the polyol.
Though gasoline is chemically complex, benzene can be measured because it is one of the few components that expresses itself clearly in gasoline spectra measured by Fourier transform infrared (FTIR) or Raman spectroscopy techniques. Some compounds, because of various quirks of nature, are much easier to spot than others.
‘Hard’ versus ‘soft’ correlations
The examples discussed thus far rely on distinct spectral expressions of chemistry that support “hard,” first-principles correlations. Unfortunately, measurements often aren’t so simple. In many situations, users want to measure properties where the spectral expression is far less distinct. In other words, the chemical signals are often buried in the sample spectrum. Fortunately, statistical modeling tools are available to help users infer what they need to know.
Instead of being hard, the correlations obtained by inferential spectrometry are considered, to varying degrees, to be empirical or “soft.” Development of statistical models may require data from a few dozen samples, or a few hundred. The modeling software extracts mathematical relationships between primary test method (PTM) values and spectra measured for samples in a calibration set (Figure 1).
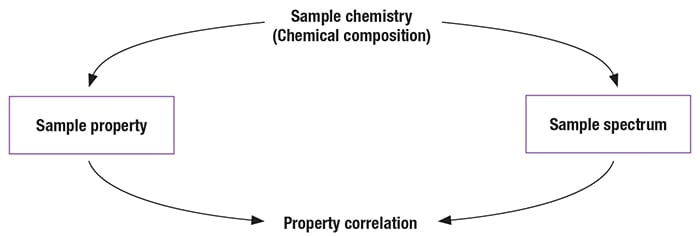
FIGURE 1. The best situation is where there is a direct and distinct relationship between the spectroscopic analysis and the specific property being measured. In such a scenario, the spectrum accurately reflects the property under investigation
That said, there needs to be caution, because the statistical modeling techniques used are extremely powerful and users can get into trouble when they assume they have a valid measurement simply because they have a correlation. Relationships can be abstract; still, chemists and engineers can and should form conclusions if they have seen sufficient evidence to indicate that components responsible for a property express themselves spectrally.
The application of such “chemical thinking” leads to the reasoned conclusion that modeling techniques can be combined with molecular spectroscopy techniques such as NIR, FTIR, Raman, and nuclear magnetic resonance (NMR) to predict octane value for gasoline (Figure 2). Each technique gives a spectrum that encodes information about the amounts of the different hydrogen-carbon structures that determine how gasoline reacts with oxygen when in burns in an engine. Modeling based on such thinking is referred to as chemometrics. Though models for octane are “soft,” they nevertheless are grounded in first principles of chemistry and spectroscopy.
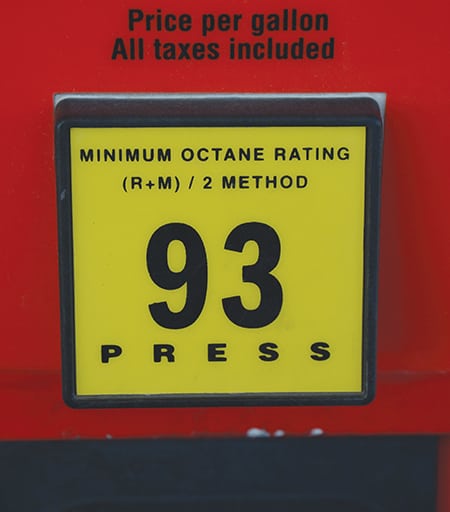
FIGURE 2. Gasoline is one of the most chemically complex products. Different batches can contain hundreds or thousands of individual components mixed in various proportions. The signature shown here represents what is coming out of a particular gasoline-blending unit today. However, tomorrow the signature will likely be different, as the component streams are tweaked, but the gasoline stream will still meet its requirements. Is it possible to determine critical characteristics (that is, octane rating or Reid vapor pressure), from this signature?
‘Circumstantial’ correlations
The same user applying the same techniques finds it possible to generate a correlation with PTM results for sulfur in gasoline at levels below 200 parts per million (ppm). Yet, just as with diesel, sulfur in gasoline can be measured by atomic spectroscopy that “counts” sulfur atoms, while molecular spectroscopy methods can only measure bonds between sulfur and other atoms. Applying chemical thinking, the user will conclude that the correlation is not grounded in first principles and is therefore unsuitable for inferring sulfur in gasoline. Apparently the correlation with ppm values for sulfur is not due to correlation with signals for sulfur. Instead, it is a circumstantial correlation rooted in unknown underlying factors.
A biochemical engineer desiring to apply NIR or Raman spectroscopy in an effort to monitor a fermentation process used to produce an antibiotic will be initially encouraged by correlations that are generated for nutrients or products in the fermentation broth. But as with sulfur in gasoline, good statistics do not guarantee good measurements.
Interestingly, both the refinery and the fermentation applications have a common characteristic: it is impossible not to get a correlation, even for chemical properties unable to express themselves spectrally. As Ronald Coase, 1991 Nobel laureate in Economics once observed: “Torture the data, and it will confess to anything.”
What’s the culprit? Data sets that yield property correlations are themselves the product of highly correlated processes. In the fermentation broth example, an increase in one compound is accompanied by increases or decreases in one or more other compounds. The refinery is an assemblage of interconnected units that produce components that can be blended to make gasoline.
Reading too much into the data
Before we dig further into the matter, let’s note two points that are both obvious and subtle. First, the spectrometer has only one purpose — namely, to measure a sample spectrum, which is simply an X-Y data array comprising intensity values across some range of frequencies.
Second, the purpose of the correlation model is to convert the raw data into a concentration or property value with specific attributes so the user is able to determine its actionability for purposes of process control. Principle among them is tracking process changes accurately and in realtime.
The subtlety is that a spectrometer is actually not an analyzer any more than a temperature sensor is an analyzer. Both merely report an intensive property of the sample, though the spectrometer’s response has many points instead of one.
This idea is well understood by process engineers who use inferential analyzers, also called soft sensors. This technology is applied in diverse industries ranging from petroleum refineries and chemical plants to cement kilns, food processing, and nuclear plants (http://www.springer.com/us/book/9781846284793). Readings from multiple sensors installed throughout a process are gathered to form a sort of spectrum, an array of intensity values. Typically, these readings come from simple univariate process sensors reporting temperature, pressure, flow, density, conductivity, current, motor torque, opacity and more. Taken together, these individual data values are then used to model a process property of interest, such as degree of conversion in a batch reactor, or the quality of a product stream from a distillation process.
For the purpose of this discussion, we have to accept one key underlying premise — the job of a spectrometer is to create a record of the sample spectrum precisely and reproducibly. Not all technology does so equally well, and that can be very problematic due to the “garbage in, garbage out” (GIGO) principle. But assuming the equipment is not the issue, the problem is what happens to the data after it is collected.
The difference between a spectrometer and an analyzer is the model. The fact is, the model is the analyzer (just as with soft sensors): it analyzes the data (the sample spectrum) produced by the spectrometer. In inferential spectrometry, the analysis is a two-step process: the spectrometer measures a sample spectrum, and then a correlation model, sometimes called a chemometric model, converts the spectrum into property predictions. This effectively turns the spectrometer into a PTM surrogate. But if pushed too far, the model may suggest a relationship that does not actually exist. The question to ask is, when has the line between hard science and wishful thinking been crossed?
Analyzing gasoline is the ultimate expression of this problem because, as mentioned before, it is the most chemically complex product we deal with on a daily basis. Given its ubiquitous consumption worldwide, it is hugely important to make accurate measurements, and any technological gain would make an enormous difference thanks to the sheer scale of production.
Crossing the line
Here is how the process works: the user wants to make some determination about a product for which, at best, the spectral correlation is soft. Over some period of time, the user applies the relevant PTM to directly measure desired property values for a set of samples. At the same time, a spectrum is measured for each sample, using whatever type of molecular spectrometer is deemed best for the application (as shown in Figure 2). When this has been done for a sufficiently large number of samples (to be considered statistically meaningful), the spectra and PTM results are combined to obtain a correlation model (Figure 3).
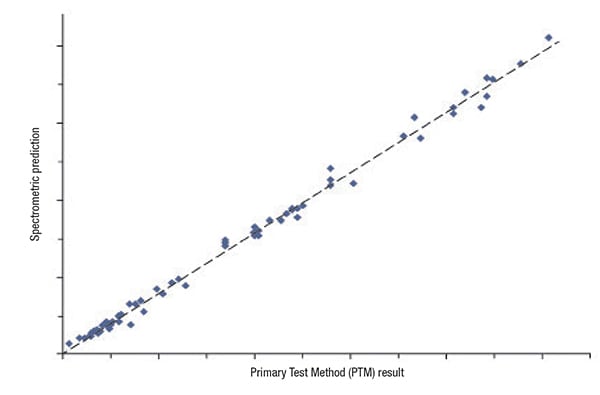
FIGURE 3. The analyzer with its chemometric model may be able to find a correlation, but does the correlation necessarily indicate a genuine cause-and-effect relationship?
At this point, the user must not only consider the quality of the correlation, but also decide if the correlation is the result of a direct cause-and-effect relationship. Does the correlation obtained truly relate to the chemistry responsible for the property? If the answer is yes, the user can implement the new testing method with confidence and reduce production costs without sacrificing product quality.
But suppose there is no direct cause-and-effect based on first principles. Because of the power of multivariate calibration techniques, it may be impossible not to get a correlation. It may be the inevitable consequence of a production process that itself is highly correlated — rather than first principles of chemistry and spectroscopy. The correlation may be due indirectly to underlying process constraints (boundary conditions), such as control over feedstocks, quality specifications and the drive to maximize profitability.
The common saying that “correlation does not imply causation” means such circumstantial correlations will not hold up. Most often, ongoing “model updating” is practiced in an effort to maintain prediction accuracy. But equally often, the user concludes that the technique simply doesn’t work. It is abandoned and the analyzer collects dust. Or, the user decides to find a different piece of equipment and is won over by a salesperson promising that a different spectrometer technology or modeling technique is the cure.
In reality, the root problem may not be either. Instead, it was simply asking both to do something not grounded in first principles of chemistry and spectroscopy — leaving the “chemistry” out of chemometrics.
Real-life examples
Spectrometric techniques are appealing because they are fast compared with PTMs, often providing spectra with an acceptable signal-to-noise ratio in well under a minute. When applied properly, they often have relatively low maintenance requirements compared with PTMs and require little or no ongoing calibration. These attributes are prime motivators today for the increased used of spectrometric technologies to make measurements of wet natural gas — measurements that are currently performed by thousands of gas chromatographs (GCs).
The main component of natural gas is methane, but wet gas can contain significant amounts of larger hydrocarbon molecules that liquefy below 100ºF, usually cataloged by the number of carbons, with methane starting at C 1. GCs are well known for being able to separate and quantify components in complex mixtures. Applied to natural gas, all of the C 1 to C 5 compounds can be resolved easily; however, things become more difficult when there are hydrocarbons present with six or more carbons. That’s because the number of possible isomers increases while the separation between their boiling points decreases. Still, isomers with identical carbon numbers can be measured as a group using GC. Thus, all C 6 isomers are grouped and measured together. The same can be done for C 7 and C 8 isomers, while C 9 and above are generally quantified as a single group. Chromatography separates and measures either individual molecules or groups of related molecules.
By contrast, spectroscopic techniques offer a mixed bag. While C 1 through C 4 compounds in wet natural gas have distinctive infrared signatures that permit them to be measured directly, the opposite is true for C 5 + compounds, due to spectral blurring (overlap). Heavy-handed application of correlation algorithms may yield tantalizing correlations, but they are limited and cannot produce GC-like measurements of heavier groups.
Returning to the world of refining and gasoline blending, two of the most critical properties are octane rating and Reid vapor pressure (RVP) as minimum and not-to-exceed measurements, respectively. Most refiners would be happy to find a way to replace the traditional testing methods for octane and RVP. The former, measured with a special, variable-compression engine, is defined as the fuel’s ability to avoid pre-ignition (knocking). This “knock engine” is a very expensive and maintenance-intensive piece of equipment. Measuring RVP with the test method outlined under ASTM D-323 involves measuring pressure in a cell at constant temperature.
In both cases, the test apparatus can be automated to provide continuous online results. But the thought of having a single device capable of measuring octane, RVP and perhaps a half dozen other properties has proven irresistible for many. So, a refiner purchases a specific spectrometer technology, obtains property correlations, and confidently begins to use the prediction values (Figure 4).
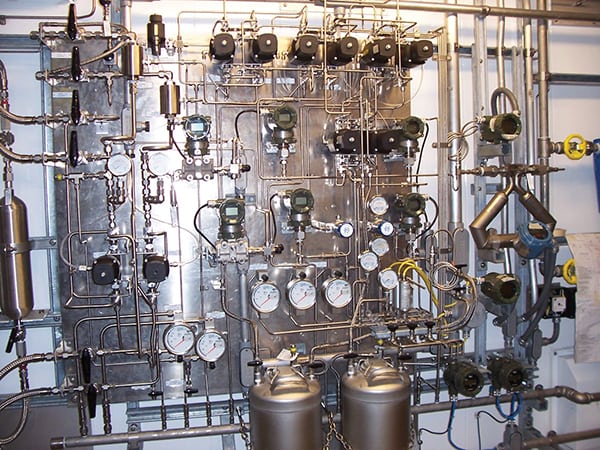
FIGURE 4. This sampling system for an analyzer installed in a petroleum refinery provides an idea of the complexity of measuring the critical attributes of gasoline
It turns out that these two properties are examples of soft and circumstantial correlations. In the case of octane, this critical attribute does not have a clearly identifiable spectral response. Instead, “octane-ness” expresses itself across a broad spectral window. Over the course of weeks and months, the refiner tests dozens or hundreds of samples from normal production using the knock engine and also records the spectrum of each. These data get fed into the computer for analysis, the goal being to produce a correlation model that accurately reports octane when applied to the spectrum of an unknown sample. The promise of eliminating the knock engine is very appealing and worth the effort.
Unlike octane, the changes in chemistry that produce meaningful changes in RVP do not register in sample spectra, locally or broadly. Yet, by following the same procedure just described for octane, the refiner still gets a correlation. Instead of inferring RVP, a circumstantial correlation has been obtained, which has poor predictive value. Here again, the adage applies: “correlation does not imply causation.” Rather, correlation is merely a confirmation that a refinery is a highly correlated and constrained system designed to produce gasoline that meets specific property specifications while maximizing profitability.
Eventually the reality becomes clear: different properties support creation of models that differ in quality, some being hard, octane being soft, and most others being circumstantial. At some point, another expert may come along and say “Your project didn’t work because you were using the wrong analyzer or the wrong algorithm. This one will do the job.”
The quest for an answer may begin again. We can’t blame the user for trying because the payoff is too big to ignore, but the ability to reliably determine key attributes of gasoline using molecular spectroscopy techniques is determined by nature and the first principles of chemistry and spectroscopy, a reality that cannot be overcome with statistics. Spectrometry can do impressive things in the right context when it is used to look for things that the technique is capable of identifying, but it should not be driven beyond the underlying science into the realm of wishes and dreams. n
Author
Marcus Trygstad is an advanced analytical technology consultant for Yokogawa Corporation of America (12530 West Airport Blvd., Sugar Land, TX 77478; Email: [email protected]). He has B.S. and M.S. degrees from St. Olaf College and the University of Utah, respectively, in chemistry, materials science and chemometrics. Through most of his career, Trygstad has been involved in chemical manufacturing and spectroscopic analytical methods, and has worked for Yokogawa for more than five years.