By leveraging advanced analytics platforms, chemical processors are revealing pump trends and insights that help increase operational efficiency
In the information age, centralized monitoring technologies offer countless benefits for the chemical process industries (CPI). Using data generated by sensors installed throughout industrial processes, engineers and operators glean valuable real-time insights into critical process parameters, equipment performance and environmental conditions, helping teams optimize plant operations and reduce capital expenditures.
Pumps are particularly critical components in the CPI that are used for material transfer in numerous chemical and petrochemical processes, including heating, reforming, refining, filtration, distillation and more. Centrifugal, diaphragm, gear and positive-displacement pumps are frequently found in these manufacturing environments, and ensuring effective operations requires deploying modern pump-monitoring technologies. This empowers plant personnel to preemptively identify potential safety hazards, prompting swift action to prevent upsets.
With effective monitoring in place, process engineers and data scientists — collectively subject matter experts (SMEs) — can proactively track pump units throughout an enterprise, minimizing failures and unplanned downtime (Figure 1). However, there are challenges to implementing effective monitoring. This article provides guidance to help processors overcome these challenges and maximize the value of their pump process data.
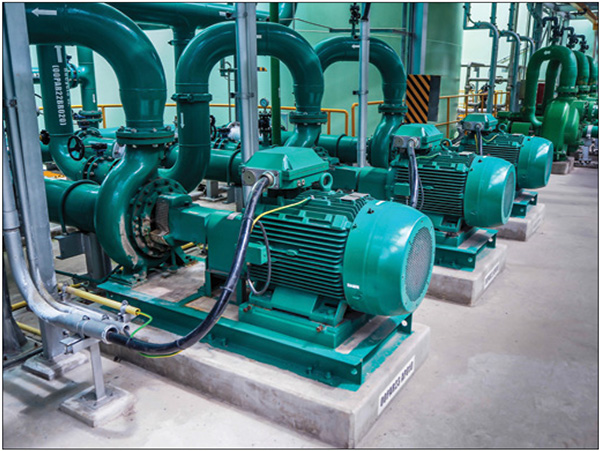
FIGURE 1. Critical data from all types of pumps can provide valuable foresight for maintenance planning, helping to eliminate unnecessary downtime
Data aggregation challenges
Manufacturers across the CPI rely heavily on sensors that generate massive amounts of data. Complex chemical and petrochemical operations in particular require data collection and subsequent analysis from numerous geographically dispersed sources, often in harsh environments, to ensure efficient and safe production.
Such operational data are often housed across various locations, such as process historians, data lakes and other databases, varying by equipment type and manufacturer. As a result, it can be difficult to standardize and deploy a centralized monitoring solution. This creates delays in identifying and addressing critical issues, which can impact production and pose safety risks.
Moreover, dispersed operations often lack the necessary tools to analyze time-series data effectively. This creates the common industrial paradigm of “data-rich, information-poor,” and despite significant investments in storage, the return on investment is minimal when data remain unused.
Historically, extracting value from monitoring systems requires specialized skillsets. In most cases, effectively deriving insights from data requires coordination between engineers (who understand the process operation) and data scientists (who are trained in coding). However, not all plant personnel possess the skillsets required to perform these tasks.
Modern analytical capabilities
Advanced analytics platforms bridge the gap between skillset limitations and operational insights, providing extensive support for connecting to and extrapolating meaning from data that may be spread among various disparate locations, regardless of the format or structure. This is accomplished with alignment algorithms and protocols that ensure data consistency and accuracy.
By automating data alignment and alleviating manual efforts, these software platforms provide significant advantages for pump monitoring, freeing up valuable SME resources and reducing the potential for human error. With a range of powerful tools for data contextualization, calculation and asset scaling, these platforms facilitate extraction of valuable insights from consolidated data without the need for coding skills, empowering users to quickly become proficient in the software, even those with limited technical expertise.
Beyond ease of use, these platforms provide advanced features for complex analysis and calculations, including predictive analytics, machine learning and statistical modeling. Additionally, extensibility features allow users to operationalize custom calculations, empowering them to not only analyze historical data, but to also predict future outcomes and optimize processes accordingly.
Automate asset organization
Advanced analytics platforms take advantage of asset-organized data structures to efficiently scale calculations across all assets of the same type. Results can be viewed in high-level visualizations, such as treemaps, with interactive drill-down functionality to access each individual asset’s underlying process data (Figure 2).
The visual layout can also be changed on the fly to view how a particular asset is currently operating, providing insight into the desired range of analytics scaling. This ensures results can be developed from the micro (individual assets) to the macro level (entire process sections or plants).
This ability to view results at various levels helps facilitate collaboration among organizational roles and management. For example, focused management reviews help operational units establish priority and generate workflow actions for operators, engineers and maintenance personnel to address issues.
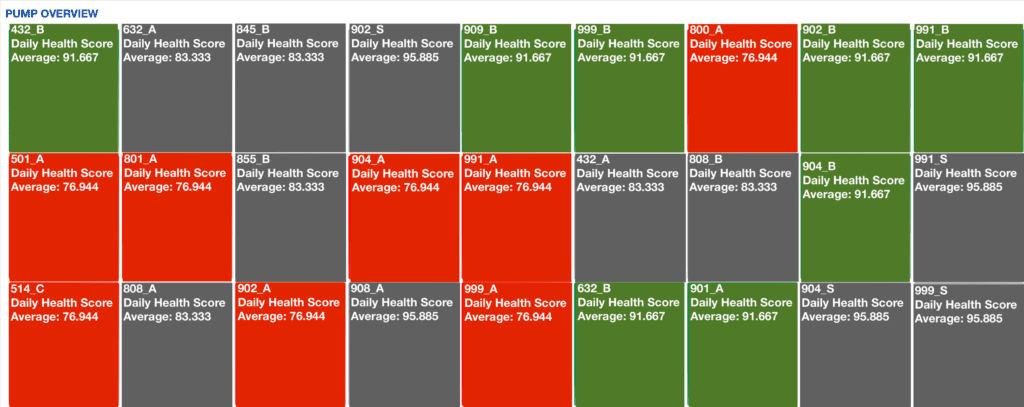
FIGURE 2. The ability to evaluate pump health using a treemap provides visibility of the performance of an entire suite of assets over time
Pump health monitoring
Pump health monitoring has become increasingly important because it leads to better methods for measuring pump performance, along with the ability to gauge pump health in near real-time. Traditional calendar or schedule-based maintenance can create unnecessary work while neglecting maintenance tasks, causing unplanned downtime, lost revenue and environmental and safety risks.
Conversely, implementing condition-based analysis to monitor pump health across fleets of pump assets leverages historical process and diagnostic data to create a predictive maintenance forecast. Doing so identifies potential degrading conditions or risks, helping prioritize maintenance activities to preemptively address pump anomalies and issues before failure occurs.
For example, by aggregating the necessary pump data from asset-management systems, process historians and vendor specification documentation, engineers can condition and correlate information, and define models for normal operating conditions. SMEs can then use data-cleansing tools to remove outliers, noise, dropouts and values from when pumps were not running, and to further condition “bad” or negative values from the raw dataset.
Based on the resultant normal operating conditions, calculations can be applied to create a histogram displaying the amount of time that a pump spends in different “mode of operation” classifications, including:
- “Good” or “poor”
- Percent of time in various “poor” modes of operation
- Percent magnitude of violation from normal during the “poor” modes of operation
This information can then be used to create a daily health score, which can subsequently be used to analyze the likelihood of pump failure (Figure 3). Displaying these results on dashboards with automatic updates to show pump health for troubleshooting potential issues and refining calculations further enables reliability engineers to perform more comprehensive root cause analyses. In the field, these types of analyses have saved companies money by avoiding lost production and unnecessary labor on each pump.
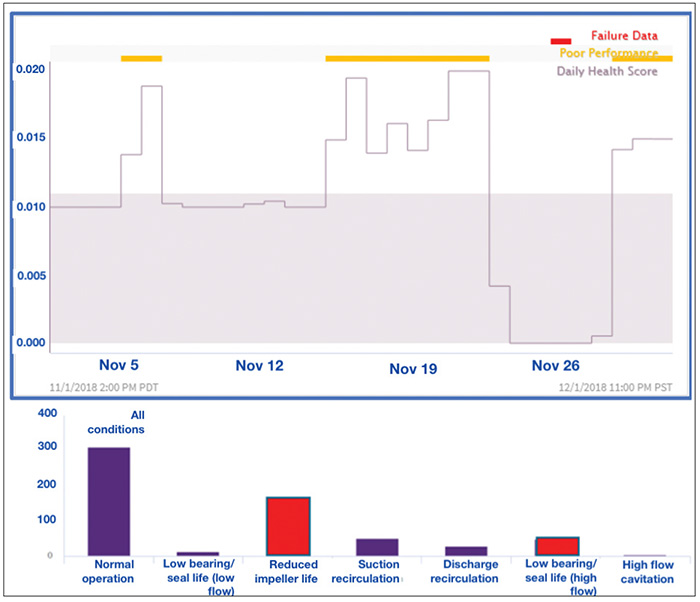
FIGURE 3. Tracking the daily health score (top) and leading failure indicators (bottom) helps operations personnel keep their pumps and processes running reliably
Example: Modeling an oil pump
Failure of oil export pumps can lead to facility-wide shutdowns, and repairs of this highly specialized equipment can be costly and lengthy in duration. Without a proactive condition-based maintenance program in place, plant personnel run the risk of unplanned shutdowns, reduced production and environmental and safety issues, leaving facilities vulnerable.
To monitor its oil export pumps, a leading petrochemical company utilized an advanced analytics machine-learning (ML) application and created an ML model using SME-identified cleansed process-instrumentation tags. To begin, the team identified historic examples of normal operation and unplanned downtime events within the application, providing a baseline and distinction for the ML software to use in its analysis.
After allowing the model to run, users reviewed results of the application’s condition detection, and determined the model correctly identified root causes of past downtime events. Next, the company commissioned the ML application to run in near-real time, utilizing current operational data to assess the risk of future failure. Built-in online diagnostics were instructive for understanding key contributors to pump failures, and to direct further investigative and troubleshooting efforts (Figure 4).
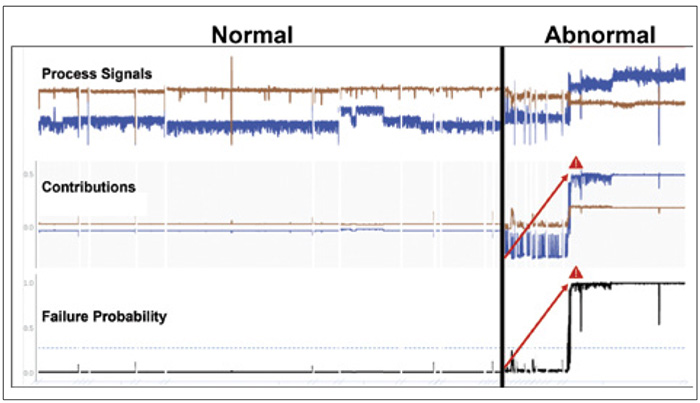
FIGURE 4. A petrochemical company used online diagnostics to automatically identify abnormal operating conditions, prompting mitigation efforts to reduce failure rates of oil export pumps
With proactive monitoring and diagnostics in place, the team improved reliability and uptime, increasing revenue by an average of $2,000,000 per day. Additionally, the diagnostic insights provided a clearer understanding of diminished or degraded performance root causes, enabling maintenance personnel to proactively service equipment prior to failure, saving time and money. The company was also able to scale this application to monitor similar assets throughout the enterprise.
Drive high-value scaleup
Advanced analytics platforms are designed to centralize data and produce previously hidden insights that can be quickly scaled across entire enterprises. These outcomes enable organizations to accelerate digital maturity and vastly bolster operational efficiency, starting with foundational assets on which processes depend, such as pumps.
By proactively identifying areas of required asset servicing using predictive maintenance capabilities, these platforms help chemical processors achieve corporate productivity objectives. While there is no standard final destination for all users, optimization efforts generate advances in product integrity, operational safety, sustainability and profitability, helping industrial companies maintain a competitive edge. ■
Edited by Mary Page Bailey
Acknowledgement
All figures courtesy of Seeq
Author
Janelle Armstead-English is the chemicals industry principal at Seeq Corp. (Email: [email protected]; Phone: 206-801-9339). She has an engineering, market research, sales and product management background with dual B.S. degrees in chemical engineering and mathematics from the University of Pittsburgh. Armstead-English has nearly two decades of experience working with various chemical and petrochemical processors, including Honeywell UOP and Praxair (now Linde). In her current role, she enjoys analyzing the ever-changing chemical and petrochemical markets and understanding the challenges around digital transformation in these industries.