Chemical companies can drive peak performance and extend the lifecycle of their assets by making the step-change from time-based to condition-based predictive maintenance
Exposed to fluctuations in global commodities markets, and typically characterized by high volumes and low margins, the downstream petroleum refining and chemical processing industries (CPI) are eschewing traditional “fail and fix” maintenance procedures in favor of new predictive digital solutions that maintain the availability of critical equipment, optimize production and reduce operational expenditure (OPEX).
This step change has been accelerated by the recent COVID-19 health crisis. Major maintenance projects with long turnaround times require large numbers of personnel to gather in one area, which is still not viewed as safe in many countries where chemical processing takes place. In addition, economic pressures have eroded margins still further, meaning companies are under pressure to maintain plant output.
Against this backdrop, employing the latest condition-monitoring and asset-performance management techniques throughout the entire asset lifecycle — driven by effective data acquisition and analysis — has never been more important (Figure 1).
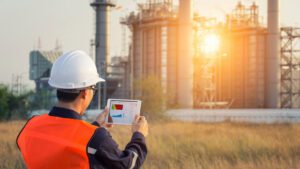
FIGURE 1. Taking advantage of advances in IIoT technologies, including predictive analytics, remote monitoring and artificial intelligence, can give users an edge in terms of avoiding failure and optimizing equipment operation
Enterprises with a coherent maintenance and asset-integrity strategy, underpinned by Industry 4.0 innovations like artificial intelligence, the industrial internet of things (IIoT) and machine learning, not only enjoy a commercial advantage; they can also demonstrate regulatory compliance, and reduce the risk of a major incident that may endanger personnel and undermine stakeholder and public confidence.
The journey from reactive to predictive maintenance
By replacing inefficient time-based maintenance processes with condition-based monitoring — and ultimately predictive maintenance, where operators not only understand the condition of core equipment, but are able to predict, for example, the number of hours of running time available, how close to the integrity operating windows they are and the probability and severity of failure over different fault mechanisms — companies across the CPI can reduce the risk of costly downtime and unplanned shutdowns.
In the past, companies tended to focus on large machinery that was deemed critical to operations, and where failure could have significant cost implications. Now, by deploying accurate wireless technologies, sensors, high-speed communications, powerful computing solutions, including cloud and edge, machine learning and advanced analytics, we can apply those same approaches to a vast range of equipment — small, medium or large rotating machines and motors, for instance — meaning that the customer can define exactly what to maintain and when to maintain it.
Consider, for example, a medium-sized petroleum refinery that has around 3,000 rotating machines driving all sorts of plant equipment. At present, many such refineries employ time-based maintenance processes that typically involve teams of subcontractors or employees going around the plant using vibration probes and other handheld sensors to understand the condition of machinery. They then go away, collect the data, prepare results and provide the information back to the plant’s maintenance and production teams. This process occupies precious resources and yet provides the condition of that piece of equipment only in the moment that it was being measured.
Carrying out this type of basic maintenance on rotating production equipment can cost upwards of $500,000 p year. Research by ARC Advisory Group reveals that preventative maintenance is only appropriate for approximately 18% of equipment in a plant. Most equipment (82%) shows a random failure pattern not determined by time. In addition, conducting preventative maintenance on the other 82% of asset types can introduce failures in themselves.
Ideally, plants can implement automatic online condition-monitoring of a variety of electrical, rotating and static equipment across plants, including electric motors, using wireless sensors to detect machine vibration and temperature anomalies that could lead to equipment failure. With today’s advanced solutions, users are not limited to receiving data from a specific point in the production process; instead, they can make more informed maintenance decisions based on continuous online data.
These types of systems can often easily be installed on existing infrastructures without extra shutdowns. Additionally, the engineering, planning, materials and installation costs are typically less than traditional wired solutions.
Below are a few helpful questions that plant engineers need to ask themselves when considering their maintenance strategies:
- Do I know the condition of my plant assets?
- Do I have sufficient capability to detect potential failure?
- Would I be able to act on fault indicators quickly enough to prevent equipment from failing?
There is no doubt that sensor infrastructure or the deployment of a predictive analytics system requires capital expenditure, but the alternative (retroactively dealing with the ramifications of failures and downtime) can be prohibitively expensive, and importantly, if companies change maintenance practices from time-based to condition-based, the return on investment can often be less than 12months.
A competitive edge
Turning digital potential into digital reality and extracting the maximum benefit from predictive maintenance means giving businesses the right information at the right time, at optimum quality.
Using multiple communication protocols and software types to extract data is slow and inefficient, and normal plant historians typically do not provide the data granularity operators need for effective analysis.
Ideally, a predictive maintenance platform will be able to improve overall operations by collecting data from field devices, gateways and programmable logic controllers (PLCs) in operational technology (OT) systems (Figure 2). It can then convert the various field protocols, such as OPC, Modbus and Profibus, into one common communication language and serve the standardized output to either the user’s or a service provider’s cloud infrastructure, while simultaneously guaranteeing no access to the field network to keep critical OT systems secure.
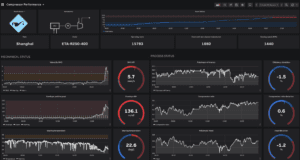
FIGURE 2. To maximize value, a predictive-maintenance platform should provide and contextualize data from myriad sources
Another benefit in modern predictive-maintenance solutions is the ability to livestream contextualized data, such as process performance information on pump reliability, for example, seamlessly from the control system or equipment to the cloud.
Analytics capabilities can then turn this critical information into actionable insights with highly customizable visuals, accessible from anywhere on any device.
Step change
A robust approach to maintaining chemical processing equipment takes the form of six distinct steps, detailed in the following sections. It is important to note that each plant has different requirements, and may already have achieved the first three steps, for example.
1. Evaluate what you have. The journey begins with gaining visibility of operations and plant assets. Typically, a company needs to understand the current performance of their assets, which may entail taking data that already exists at the plant level and visualizing it using an IIoT platform and/or a distributed control system (DCS). An operator can marry this data with information technology (IT) and maintenance-management system level data for a better view of maintenance histories paired with plant performance. In this situation, the key is often capturing data that has been previously lost and integrating it into the IT domain safely and securely.
2. Identifying gaps. Step two involves understanding information gaps and remediating them by converting traditional motors, pumps and mounted bearings into smart, wirelessly connected devices using smart sensors that can typically deployed in minutes. These measure critical parameters from the surface of the equipment, which can be used to gain meaningful information on the condition and performance of the machine, enabling users to identify potential faults within their system, and reduce risks related to operation and maintenance.
3. Begin the transition. Stage three would implement an advanced software or IIoT solution to help users begin the transition to predictive maintenance by gathering and presenting real-time plant condition data from disparate systems in one dashboard, and running the data through equipment specific asset monitors making it easier for personnel to analyze it and make informed decisions.
4. Advancing the predictive model. Step four is about using more advanced predictive algorithms and combining a heuristic/physical modelling model with a data science-based approach — to better understand the health of equipment and its performance characteristics and adapt the maintenance strategy accordingly. Consequently, this step should employ machine learning and model-based analytics to compare actual equipment performance against expected performance at different ‘good’ operating points. Diagnostics through predictive analytics can be used to detect problems early and express data primarily in the failure mode and effects analysis (FMEA) format familiar to all reliability engineers, enabling them to act before faults or failure occurs. An online system should constantly check the current plant state. Using trending and forecasting algorithms, the system can then inform the user when the machine health is likely to deteriorate further, potentially to failure.
5. Enterprise planning. The penultimate step is to use this reliability information and statistics to plan and expand across the enterprise, linking optimized strategies to other workforce management systems or overall enterprise management solutions in order to provide the right solution to optimized maintenance for each group of assets or the holistic system ISO 55000 is a common international asset-management system that is standard across multiple industries. From here, the goal for maintenance strategies is to progress to a prescriptive maintenance regime, where advanced analytics will not only direct maintenance operations, but act on the information; for example, by raising works orders for a technician and ordering parts required for the operation, and informing relevant business users of the information that is appropriate to their role
6. Establish an overarching culture of reliability. The final step involves adjusting operations and establishing a reliability culture where everybody recognizes their personal responsibility, just as the importance of safety is widely recognized. Companies must not neglect the role of people and process, no matter how advanced the technology deployed. ♦
Edited by Mary Page Bailey
Author
Will Leonard is asset performance management product manager, Energy Industries at ABB in the U.K. (Email: [email protected]). He leads ABB’s portfolio of APM software and services that help energy operators improve the reliability and availability of physical assets while minimizing risk and operating costs. The portfolio includes condition monitoring, predictive maintenance, asset integrity management, reliability-centered maintenance, and involves technologies such as asset health data collection, visualization, and analytics