Presented here are some essential steps to create useful information and drive real results in your operations with data analytics
As technology continues to advance, it’s no surprise that chemical producers are looking to leverage new solutions and rethink business strategies. And the chemical process industries (CPI) are no exception.
Historically, growth strategies in the CPI have been based on mergers and acquisitions, according to the 2018-19 Chemical Trends report by PWC. The same report, however, shows the number of deals dropped significantly in recent years.
Today, chemical producers are looking beyond their traditional growth strategies for new profitability and asset utilization opportunities – such as turning to solutions that rely on digitalization techniques, including analytics.
The true value of industrial analytics is in the results they can deliver in wide-ranging industrial sectors. For instance, at a brewery, analytics delivered a 60% boost in throughput. A pet food producer realized more than $800,000 in annual energy-cost savings from implementing analytics. For a cosmetics plant, industrial analytics delivered a 90% reduction in line stoppages. An oil-and-gas producer reduced operational costs by approximately $30,000 per year by implementing analytics. And a petrochemical company reported improved product consistency by 35% when applying analytics capabilities.
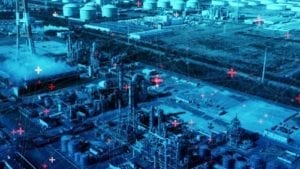
Industrial plants create an immense number of data points. Contextualizing these data can bring practical insights that improve operations
Without a doubt, the ability to not only collect raw data, but also convert the data into useful information for workers, is essential to staying competitive. But many people may be unsure of how to bring analytics to their operations — many find the process daunting, lack domain expertise and resources and don’t know where to start. Or, they may have a goal to deploy analytics, but no defined strategy in place. Fortunately, implementing analytics doesn’t have to be a mystery or an overwhelming task. Those that have already made the journey can provide key lessons for how others can begin their own analytics journey.
Core elements
Industrial analytics is built around four core elements, described in the following sections.
People. Data scientists are vital to deploying analytics. But the everyday use of analytics should not hinge on these specialized workers. Today, new analytics technologies work almost as an “in-the-box data scientist” to structure users’ data upfront. Then, non-data specialists, such as chemical engineers, R&D scientists and operations teams, can freely access, manipulate and analyze key data, such as real-time production key performance indicators (KPIs), such as throughput and quality, operator effectiveness and plant safety data. This “self-service” analytics approach allows almost any worker in a company to use data to solve various issues, leading to lower mean time to repair (MTTR), better first-run quality and improved asset utilization.
Data. According to a recent industry study, the key operational and data challenge for chemical producers is the ability to integrate data across multiple systems, with availability and access to the data also a top concern. To combat this challenge, it is critical that companies first identify what data they want to collect and where it will come from before beginning their analytics journey. You may collect some data from your people. But most of it will come from connected technologies known as the industrial internet of things (IIoT). These technologies may include process instrumentation, analyzers, drives and motors, skid-level components and plant-level platforms like distributed control systems (DCS), safety instrumented systems (SIS) and other software systems, including laboratory information systems (LIMS), enterprise resource planning systems (ERP) and computerized maintenance management software (CMMS).
Connectivity. Analytics requires seamless connectivity across a plant floor. This may require a company to unify any disparate systems that have created islands of information or cannot talk to each other. It also means that you need to provide the bandwidth for both current and future traffic needs. Standardizing your plant-floor network on a technology like EtherNet/IP can help to achieve real-time control and information. Pre-engineered network products and services like industrial data centers or infrastructure-as-a-service (IaaS) offerings can also reduce your network design and configuration time.
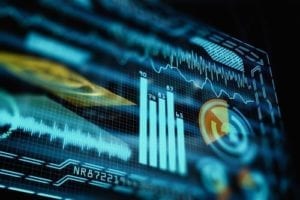
A robust analytics software can highlight specific anomalies in data and help to troubleshoot operational issues
Analytics software. Chemical producers should look for analytics software that delivers the best value to their organization. The software’s most basic job is to add context to data. Context allows users to combine and compare different data to get a deeper understanding of operations. Instead of simply pulling a temperature reading from a chemical unit operation, for instance, data contextualization can provide that reading at a certain time, as well as certain surrounding conditions, asset context, raw-material properties and human interactions during a specific shift or timeframe. This helps better understand KPI behavior with insights leading to near real-time actions to help maintain safe and profitable operations.
An analytics software product can and should also do much more than contextualize data. For example, it should allow users to drill down into specific analytics to investigate anomalies or troubleshoot issues. It can even use machine learning to monitor operations and trigger automatic control adjustments if a process falls outside allowable parameters. An example of this functionality would be utilizing “golden-batch” KPI tracking to indicate that a batch is drifting out of specification to help operators address it before an off-spec product is introduced.
Underlining the importance of software implementation is an example from the chemical manufacturing sector. Shanghai SECCO Petrochemicals Company Ltd. (SECCO) wanted to maximize its polymer production rate and improve product consistency while maintaining specifications. To help meet these goals, the company implemented a model predictive control (MPC) analytics solution. The MPC software helped SECCO to more quickly adapt to changing market conditions and customer demands. MPC analytics is used to continuously assess current and predicted operational data, compare it to desired results, compute new control targets to reduce variabilities and help operate within equipment constraints to improve process performance.
After deploying the solution, SECCO quickly maximized its production rate and improved product quality. Specific results include the following:
- Increased production capacity by 4%, on average
- Improved product consistency by 35%, on average
- Reduced reactor variability by 20%
This analytics solution helped SECCO realize major return on investment (ROI) just four months after implementation, and it continues to help keep costs down and improve revenue.
Where to Begin for a Successful Journey
The four elements outlined above should form the foundation for building and executing an analytics platform. Additionally, there are key decisions chemical producers will need to make early in their journey to maximize the success of an analytics approach long-term.
Solve business needs
Your analytics investment can pay for itself, but it is important the investment is tied to specific business outcomes and is not merely about the technology.
In fact, a Deloitte chemical Industry 4.0 study found it best for chemical producers to think through opportunities modern solutions can offer, then deploy the technology based around those potential outcomes, such as energy usage or productivity/product throughput improvements. Next, you can determine the productivity boost or specific energy reduction required to meet your specific goal and identify return on investment (ROI). Then, companies can use that ROI to fund subsequent analytics initiatives, like machine learning or augmented reality.
For example, many chemical producers are operating on legacy DCS and batch systems — often these systems may be 20-30 years old. Due to the age of the systems, many fundamental processes are manual, creating human error. How can these producers use analytics to improve these operations and empower employees electronically? In this case, the producer would choose analytics to improve operations driven by a decrease in human error. Analytics can be applied to provide data in a way that that shows operators exactly what action to make based on alarms and historical trending.
Collaborate with partners
Existing industry partners can play an important role in your analytics strategy. On-machine analytics from your original equipment manufacturer (OEM) or licensor, for example, can help maximize the performance and durability of your process assets. With just a gateway device installed on a machine or skid, analytics can be delivered via cloud-based applications to help drive better asset utilization.
Get the support you need
Finally, make use of available services and resources. They can help you get the most value from your analytics deployment and alleviate whatever challenges you’re facing.
Reference architectures, for example, can help you design and implement your network upgrade. The chemical industry is struggling to overcome human capital shortages, so training and certification programs can equip your workers with the skills they need to design, deploy and oversee a secure information architecture. And connected services can help you deploy analytics, or even take on key roles for you, such as remote monitoring of operations. Many manufacturing companies have very specific pieces of equipment that require specialized support. In some cases, building that support internally is more expensive than actually having that support externally.
It has never been easier or more cost-effective for chemical companies to deploy analytics and modern digital solutions. The foundation needed to get started is well established. New technologies can put the power of your production data into the hands of the workers who need it most, helping your company make better business decisions and identify new growth strategies.
Given the opportunity that analytics represent, deploying analytics shouldn’t be a question of where to begin but, rather, when can I get started? ♦
Edited by Mary Page Bailey
David Stonehouse ([email protected]) leads Rockwell Automation’s Global Consulting Services Team. He has over 25 years of experience as an operations leader and management consultant. Prior to consulting, he led Kohler’s supply chain operations for the Americas. Stonehouse has a B.S.Ch.E. from Queen’s University (Canada) and an M.B.A. from Western University (Canada).