In a data-overloaded environment, companies may struggle to fully reap the benefits of Industry 4.0 technologies. Understanding data utilization can help transform process datasets into decision-making assets
The chemical process industries (CPI) have been realizing for some time that information is of strategic importance for the survival of any organization. Colloquially, data have been called a new natural resource [1] — every part of an organization is making decisions daily, based on data they collect, process, analyze and review. The global pandemic has further driven the awareness of digital transformation for any company, and manufacturers are no exception to this. The rise of Industry 4.0 technologies has further driven this trend, and with more and more data available, organizations are struggling to reap the benefits of these data. At the same time, the industry acknowledges that poor engineering information can have real and significant impacts, but little research has been done to quantify this. Among this mixture of promising potential and data overload, companies are struggling to define where and how to start. This article shines a bit of light on how an organization can make progress in its quest to transform its vast volume of process data into a decision-making asset.
What does data mean for CPI firms?
CPI facilities collect massive amounts of data. One way to describe the types of data being recorded is to look at the different levels of the manufacturing execution system (MES). The MES is the culmination of tools and technologies used at different levels to manage the operations of the facility. Often, the MES is also displayed as an automation pyramid. The left side of Figure 1 shows an example of an automation pyramid. Considering each of the levels illustrated in Figure 1, let us examine the different types of data being collected.
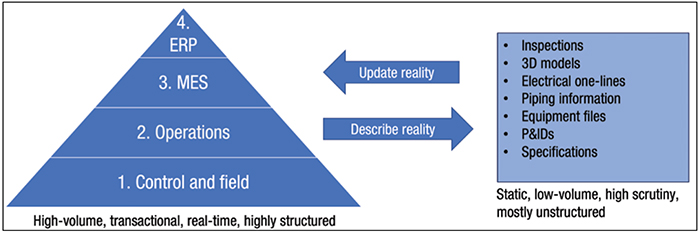
FIGURE 1. This sample automation pyramid includes the hierarchy of data sources feeding into the enterprise resource planning (ERP) system and manufacturing execution system (MES), as well as supporting datasets
Level 1: Control and field. At this level, the input from the field is generated by sensors and equipment used in the field. Temperature, pressure, rotational speed, valve status and many other variables are generated at very frequent increments. The inputs generated at this level are provided to the second level in the pyramid.
Level 2: Operations.The second level provides low-level automation in the form of programmable logic controllers (PLCs), which ensure basic controls for individual systems and operate and respond at very high speeds.
Level 3: MES.The third level is the MES level, often executed in the form of a distributed control system (DCS) or supervisory control and data acquisition (SCADA) system. There are obvious differences between these systems, but for this article, their function is the same: to provide overarching control of the facility across multiple systems. Ref. 2 provides a good overview of the systematic differences.
Level 4. Enterprise resource planning (ERP). The ERP level provides integrated resource planning and controls the planning and resources required to generate the desired production.
Each lower level can produce data that can be used by a higher level to create a more integrated system. Research has been conducted on the importance of this integration. According to these findings, to reap the full benefits of Industry 4.0 technologies and effectively utilize digital twins, data must be aligned at all levels [3].
A second description of the data being generated is the supporting data used to ensure safe and compliant operations — for example, piping and instrumentation drawings (P&IDs), specifications, inspections, environmental reports, work processes for management of change (MOC), training records, and many others. Where the MES generates vast amounts of transactional and real-time data, the supporting data are more static. With the data being more static, the change controls put in place on these data are rigorous enough to ensure the safety and compliance of the facility.
When both types of data are presented in one overview, it becomes more evident how they interact and the importance they both play in the day-to-day operations. This is displayed in the right side of Figure 1, which shows an overview of the two important datasets. There are many interaction points, and operators, engineers, planners and project managers will use both datasets daily to operate the facility. In an ideal world, both datasets are aligned, represent reality and support the operation. The truth is very often different — companies may be struggling to keep the data updated both vertically and horizontally across both datasets. During the 2012 Offshore Technology Conference (OTC), it was stated that there is often a 12-month lag time between the real world and all information systems being correctly updated [4].
The impact of poor data
Previously, the types of data that are maintained and created as part of the operation for a production facility were described. With a general feel for the struggles to maintain this information, it is important to ask, why does it matter? Amongst information-management experts, there is a good sense that poor engineering information has an impact. However, when researching this topic, it is difficult to quantify the impact, as research on this topic is very limited. The usual suspects relate to safety, compliance, efficiency, improving equipment reliability and so forth. The following sections discuss some of these areas in more detail to see if there is a business case to be made before digging in further.
Trust — 50% or worse. From a limited poll during a live event, amongst process manufacturing companies, 50% of the attendees rated the trust level of their information at 50% or worse. At a minimum, this means that a field-check is required, and multiple systems would need to be verified, before committing changes to critical information [5].
Perceived reliability — 60%. From a study conducted in 2016 amongst nine petroleum production facilities and 133 interviews, 60% of the group reports that communication and access to information, combined with the efficiency of the tools available have a large influence — in a negative way — on the perceived reliability of the equipment [6].
Safety and incidents — 86%.When an incident (or near miss) happens, 86% of those surveyed at a live event for process manufacturers believed that poor, missing or not timely information was a large contributing factor to the incident [ 5].
Cost — 1.5% of annual revenue. From research performed by ARC Advisory Group (Dedham, Mass.; www.arcweb.com), it was concluded that the cost of poor asset information can be upwards of 1.5% of annual revenue for an organization. Throughout the study, these numbers have been validated against other known studies to contrast, and compare and validate the outcome of the study [7].
Cost — $15.8 billion per year. From a well-known report produced by the National Institute of Standards and Technologies (NIST; Gaithersburg, Md.; www.nist.gov), it was estimated that the inadequate interoperability of information is costing the capital facility industry a staggering amount yearly. Although this study was performed in 2004, reviewing some of the more recent research shown above the presented number still seems relevant [ 8].
Cost — 10% of the operating budget.From a study in 2019, it was concluded that up to 10% of an annual operating budget could be lost because of poor engineering information. Many variables are used, and depending on the specific situation these numbers will vary a lot. However, the range of potential cost is very significant for any asset that provides a solid start for a business case [9].
With the arguments above, it should be easy to convince leadership to invest in improving the quality of information. The reality is very different, as most of these figures represent soft or indirect savings and results that require not only a system, but also organizational changes in culture, structure and work methods to fully reap the benefits. The most recent research from Volk and Coetzer has produced a taxonomy showing an overview of the cost involved with poor engineering information for a facility [9]. Figures 2 and 3 show an overview of the defined taxonomy.
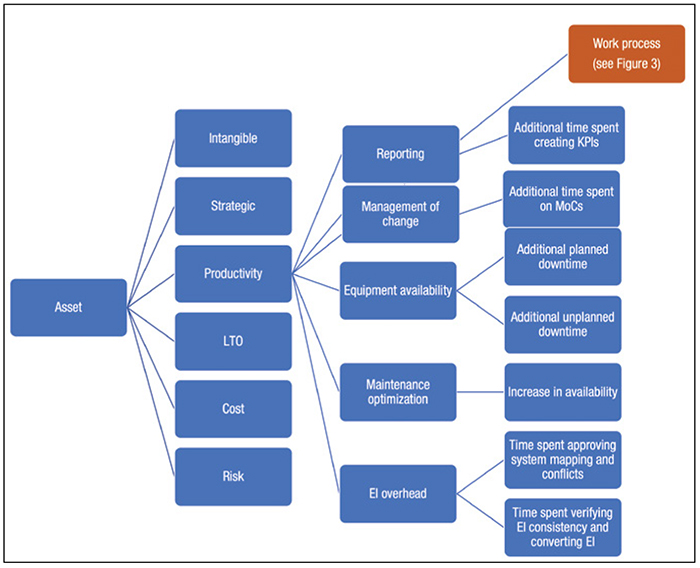
FIGURE 2. This taxonomy gives an overview of the potential costs associated with poor engineering information [9]
Executives have grown weary of large information technology (IT) projects that take a lot of time with limited hard benefits. While improving data quality sounds a bit mundane, coupling data quality with digital transformation and improving how business is conducted will have a higher chance of achieving the executive agenda.
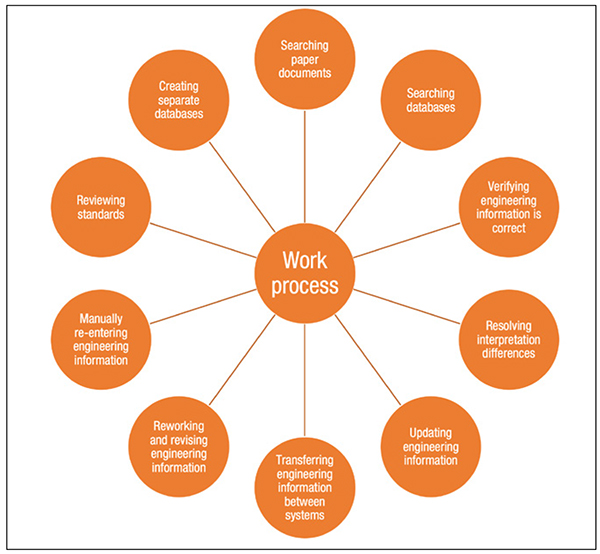
FIGURE 3. If an organization is dealing with poor engineering information, there are many work-process activities that may require significant additional time
To provide some practical guidance, Frank et al., describe stages of Industry 4.0 implementation patterns, where vertical integration of information systems is one of the elementary steps to allow a company to access more advanced scenarios. In the first stage, most companies focus on vertical system integration, energy management and traceability of materials. The second stage will focus on automation and virtualization. The last stage will focus on making the production process more flexible [10].
From different points of view, we now see that the quality and integration of data are essential for not only improving the current operation, but also for embarking on any type of transformation project. As the journey continues, establishing the meaning of data quality is an integral part of this quest.
What is data quality?
Throughout this article, the terms data and information have both been used. Although these terms are used interchangeably from time to time, there are clear differences. Amongst academics, similar definitions emerge. The word “data” is defined as a discrete and objective description of events — for example, a temperature reading for an instrument in the facility or a listing of dates for approved changes to the facility. Information is defined as data that has been transformed to add value in a specific context by condensing, categorizing and other means to support the decision-making process [11]. A good example in this sense is to use the database with recorded changes (MOC) to find trends related to equipment. A valid question to ask is: are specific pieces of equipment more prone to failure compared to others? The answer could influence buying and replacement decisions.
Another popular buzzword in this discussion is “big” data. The obvious point to look for big data applications is along the lines of the automation pyramid with technologies such as predictive maintenance. In the context of big data, there is often a reference to the “five Vs,” as follows:
- Volume — Describes large amounts of data either stored or in transit
- Variety — Speaks to both structured and unstructured data and a wide variety of data sources
- Velocity — Describes the speed at which the data volume increases, often at a very high level
- Veracity — Relates to the accuracy and quality aspect of the data
- Value — The value created by the data for the organization [12]
Big data is not a state of being that one can achieve, but rather more of a framework for tools and methods used with varying data sources to deliver value from these complex and large data sources.
While combining different data sources at large volumes with varying complexity across structured and unstructured data is at the core of a big data project, it is easy to see how important it is to have data aligned both horizontally and vertically. For many facilities, through the years, data have been managed differently, with the result that a simple equipment tag is often labeled differently in all the different systems. Searching for information on a specific pump becomes that much more difficult, and users must navigate between asset management, drawings and SCADA systems to find the information they are looking for. Aside from system requirements that force inconsistencies, information is often transferred in a manual way, which compounds the underlying issue.
Although there is a logical inclination to look at the automation pyramid for big-data applications, using asset-management systems and document-management solutions can provide a trove of useful information. Utilizing process analytics, it is now possible to optimize business processes, while learning from real-world application usage. This capability can help to debottleneck common work processes.
Finally, data are classified into two major types — structured and unstructured. Structured data are stored in databases, such as historians, ERP systems, document-management systems and so on. Structured data are often used for reporting and analytics. On the other hand, there is unstructured data, consisting of documents, drawings, specifications, emails and so on. Due to the unstructured nature, it is much more difficult to gain knowledge from unstructured data. It is often anecdotally referenced that any given enterprise has about 80% unstructured data and about 20% structured information.
Armed with a better understanding of the kinds of data available, let us review some of the quality aspects that are essential to any dataset. Whether they are used for small-scale integration or a big-data initiative, these aspects remain valid.
Data quality can be, in simple terms, referred to as fitness for use. Wand and Wang [13] have used commonly cited dimensions from literature to describe data quality. Table 1 lists the most cited dimensions that describe data quality. Not all of these dimensions are applicable for all data sources, but this table can serve as a good means of assessing data quality. Each of these aspects can be different for a given data source, and it is up to the company to define what these levels of quality mean for the given dimension. As a practical example, let us assume the information presented on the title block for a drawing. When referring to the completeness dimension, a company could define that a complete title block should contain the drawing number, revision, revision date, approver, reviewer, editor and other elements of information. For the format dimension, the company could define that for each P&ID, there is at least a PDF version and an associated native (for instance, a CAD drawing) version of the document. Several industry standards are available that can serve as a starting point in this regard, such as ISO 15926, CFIHOS and PIP standards.
What are maturity assessments?
At present, it is close to impossible to look at any business process that is not dependent on information and communications technology (ICT) in some shape or form. To what extent this process is optimized and aligned with the strategic goals is a different question and relies on a continuous improvement process and assessment of the current position against internal and external goals, laws and requirements [14].
As business leaders seek to make improvements, they need management instruments and methods to drive their agenda. This is where maturity models come into play — using the model for evaluation of the current state, as well as providing direction for improvements [15]. For a given business area (such as information quality), a maturity model allows an organization to measure itself against a given set of criteria.
Depending on the design of the model, this measurement takes the form of level descriptions, where each level provides an established list of criteria to achieve the level. Alternatively, measurements can be taken based on a Likert scale (bipolar) approach where a detailed question will guide the respondent in answering, using a pre-defined scale. In both cases, the lower score represents an area that is less developed, where the higher scores represent well developed or optimized areas. The scoring then presents a potential roadmap for a given domain as it progresses to higher levels on the scale.
ICT maturity models found their origins from the need to better manage software processes, during the late 1980s and 1990s when many ICT projects were over budget and excessively late, while often not delivering what was promised. The Software Engineering Institute, with support from Mitre Corp., developed the first process maturity model, which later evolved into the capability maturity model or CMM [ 16]. Figure 4 shows the different maturity stages often used in maturity models.
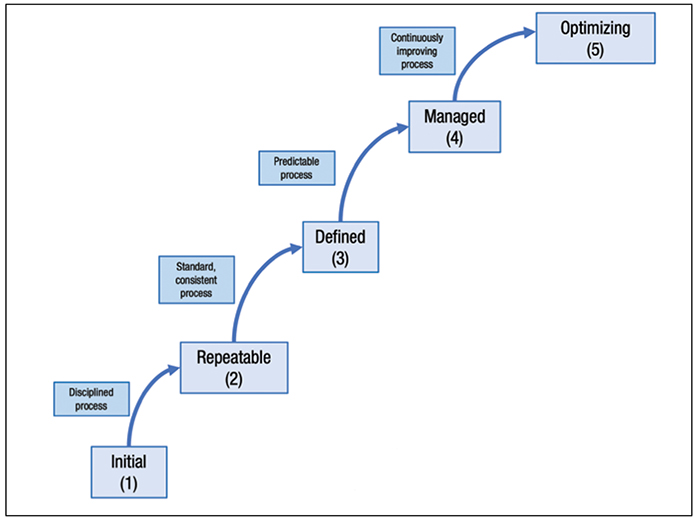
FIGURE 4. The staged format of the capability maturity model (CMM) can help to guide procedural development, but users should practice caution to ensure the model takes into account process specifics [16]
At present, maturity models are available for an almost unlimited number of topics. Performing a quick search on the internet will show maturity models for digital transformation, Industry 4.0, project management and almost any other topic one can think of. Although these models are widely credited for their usefulness, there is also a cautionary tale. The quality of the model and associated assessment is highly dependent on the specific application. To use a generic document-management maturity model about engineering information will leave out important elements. There are also many maturity models created that barely pass the academic stage. In selecting a model and assessment, selecting a widely used model will ensure higher accuracy and relevance to your organization.
Avoiding problems
While maturity assessments are a great management tool, it is important to define the proper scope and define the expectations for what the maturity assessment project will deliver. One of the key elements in digital transformation is to experiment and fail fast. While a maturity assessment will validate and provide direction, it is key to identify practical goals that can be resolved within a foreseeable timeframe and build success. Organizations currently use the term “business agility,” meaning that there is a desire to address problems quickly as the business evolves in an ever-changing landscape. The purpose of an assessment is that it will provide a roadmap whereby identified improvements can be executed, contributing to the goals of the company.
With the result of an assessment available, it is now time to translate the measurement into executable goals. One methodology is to discuss the different areas of the assessment and rate these areas on a priority scale, taking into account the company goals, budgets and available resources. Based on the selected areas (for instance, deliverables management) the organization then uses the following goal methodology (SMART) to define the improvement project [17]:
- Specific — What the project will accomplish with a very specific goal
- Measurable — How the success of the goal can be measured.
- Assignable — Who in the organization (internally or externally) will be responsible for this goal
- Realistic — Why this goal is attainable
- Time-related (or time-bound) — The timeframe for when the goal is to be completed
The combination of the SMART Goals, combined with the outcome of the assessment, provides management teams with the instruments to measure the current status, but also any future progression. The defined goals provide a method to execute specific projects that will raise the level of maturity on the topic of data quality. ■
Edited by Mary Page Bailey
References
1. Tremmel, P.V., IBM Chief Gets Standing Ovation at Commencement, Northwestern Now, June 2015.
2. Anderson, M., What are the Differences Between DCS and Scada?, Realpars B.V., Oct. 2019.
3. Weber, C., Königsberger, J., Kassner, L. and Mitschang, B., M2DDM – A Maturity Model for Data-Driven Manufacturing, Procedia CIRP, vol. 63, pp. 173–178, 2017.
4. Noller, D., Myren, F., Haaland, O., et al, Improved Decision-making and Operational Efficiencies through Integrated Production Operations Solutions, Offshore Technology Conference, May 2012.
5. Kinsmen Group, Magic of AI & Machine Learning for Engineering, Operations & Maintenance, Webinar, 2020.
6. Antonovsky, A, Pollock, C., and Straker, L., System reliability as perceived by maintenance personnel on petroleum production facilities, Reliab. Eng. Syst. Saf., vol. 152, pp. 58–65, August 2016.
7. Snitkin, B.S., Mick, B. and Novak, R., The Case for Developing an AIM Strategy, ARC Strategies, July 2010.
8. Gallaher, M.P., O’Conor, A.C., Dettbarn, J.L. and Gilday, L.T., Cost Analysis of Inadequate Interoperability in the U.S. Capital Facilities Industry, NIST Advanced Technology Program, pp. 1–210, August 2004.
9. Coetzer, E.O. and Volk, P.J., A standardized model to quantify the financial impact of poor engineering information quality in the oil and gas industry, South African J. Ind. Eng., vol. 30, no. 4, pp. 131–142, December 2019.
10. Frank, A.G., Dalenogare, L.S. and Ayala, N.F., Industry 4.0 technologies: Implementation patterns in manufacturing companies, Int. J. Prod. Econ., vol. 210, September 2018, pp. 15–26, 2019.
11. Davenport, T.H. and Prusak, L., “Working knowledge: How organizations manage what they know,” Harvard Business Press, January 1998.
12. Reis, M.S. and Kenett, R., Assessing the value of information of data-centric activities in the chemical processing industry 4.0, AIChE J., vol. 64, no. 11, pp. 3,868–3,881, May 2018.
13. Wand, Y. and Wang, R.Y., Anchoring Data Quality Dimensions in Ontological Foundations, Commun. ACM, vol. 39, no. 11, pp. 86–95, November 1996.
14. Becker, J., Knackstedt, R., and Pöppelbuß, J., Developing Maturity Models for IT Management, Bus. Inf. Syst. Eng., vol. 1, no. 3, pp. 213–222, June 2009.
15. De Bruin, T., Rosemann, M., Freeze, R., and Kulkarni, U., Understanding the Main Phases of Developing a Maturity Assessment Model, ACIS 2005 Proceedings, p. 11, January 2005.
16. Paulk, M.C., Curtis, B., Chrissis, M.B. and Weber, C.V., Capability Maturity Model, Version 1.1, IEEE Softw., vol. 10, no. 4, pp. 18–27, February 1993.
17. Mind Tools, SMART Goals: How to Make Your Goals Achievable, March 2016.
Author
Edwin Elmendorp is an information architect at Kinsmen Group (9595 Six Pines Dr, Ste 8210, Houston, TX; Phone: 484-401-9089; Email: [email protected]), a team of information management specialists working with numerous oil, gas, pharmaceutical and utility companies. Elmendorp has close to 20 years of consulting experience related to engineering information management. After initially graduating as an electrical instrumentation engineer, he added a computer science degree and recently graduated cum laude with a M.S. in business process management and IT. Elmendorp has worked with many owner-operators to digitally transform how companies manage their information assets spanning many different software solutions.