Demystifying Industrial AI Agents: What we can learn from Iron Man
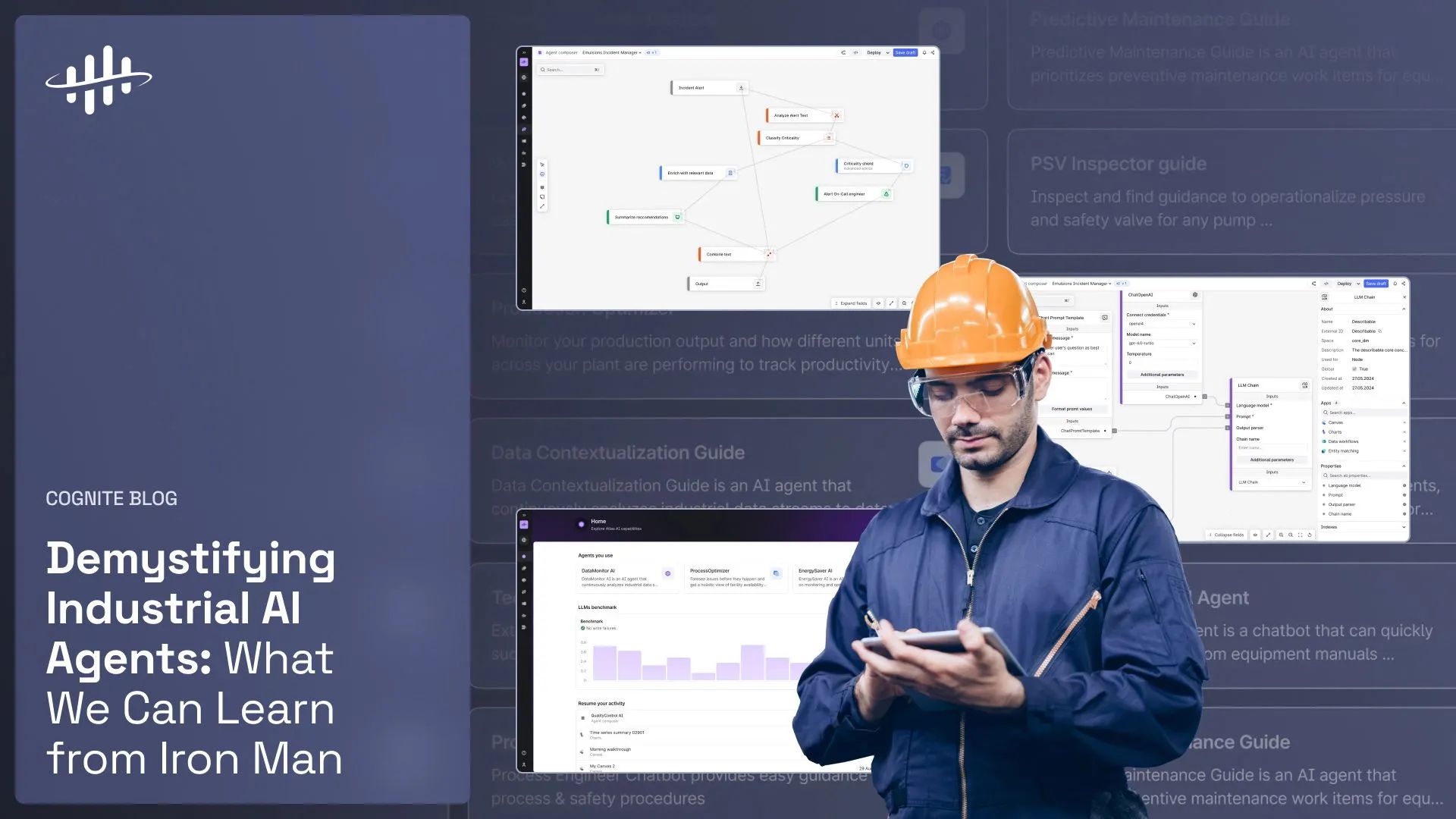
With all the hype around generative AI for industry, it seems as if there is a new buzzword almost every day. The latest? “Industrial Agents.” But is it really a buzzword? Or rather, a more accurate depiction of the actual endgame of data & AI for industry?
Few standard definitions of this term exist yet for industry, but this one is close: “An industrial agent is an agile and robust software entity that intelligently represents and manages the functionalities and capabilities of an industrial unit.”1 Put more simply, industrial agents perform specific tasks in a human-like manner when trained with the right data and when using the right AI model and capabilities.
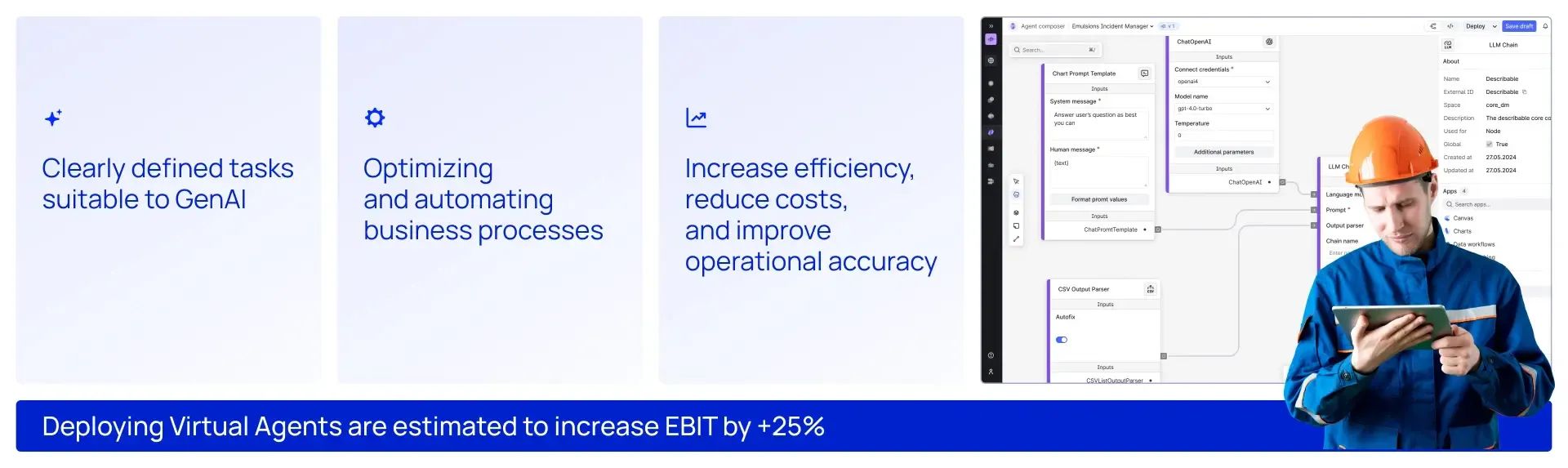
The operational co-pilots that everyone is talking about or the chatbot you use when trying to rebook your flight are all types of AI agents. They aim to automate or simplify a specific, constrained workflow to improve the user's productivity. But the agents of today that use limited pre-programmed logic are no match for the Gen AI-based agents of the future.
If we take inspiration from the movies, we’re getting closer to Iron Man’s “Jarvis” assistant - a supercharged intelligent virtual agent that communicates via voice commands and helps Iron Man do his best work. While we’re a far cry from this type of omnipresent, cross-functional intelligence (due to the no-risk, high-reliability nature of industrial operations), the technical building blocks and terminology exist today to start developing specific industrial agents for particular operator domains.
For decades, industrial operators have been trying to use data and AI to optimize production, minimize outage risk, streamline production, and make smarter daily decisions. They’ve used physical and machine learning models to classify types of asset failure, natural language processing to search for information, and now LLMs to analyze and summarize data and make recommendations. However, with the exception of robotic process automation (RPA) for back-office functions, the impact on factory-floor operations has been underwhelming.
Why is this? Well first, as with any new paradigm, it’s taken a bit of time to learn and understand what’s required from a technological and process perspective. For example, in the early phases of generative AI, circa 2023, the idea was to go straight to a “Jarvis-based future” with few general agents, with broad objectives trained on large sets of data structured and unstructured data. But this made it difficult to trust and repeat the results due to the inherent hallucinations and other limitations of generative AI.
Fast forward to today: the more realistic scenario is the orchestration of many specific virtual agents, trained on smaller, secure, relevant data sets, designed with intuitive UX to definitively improve workflows.
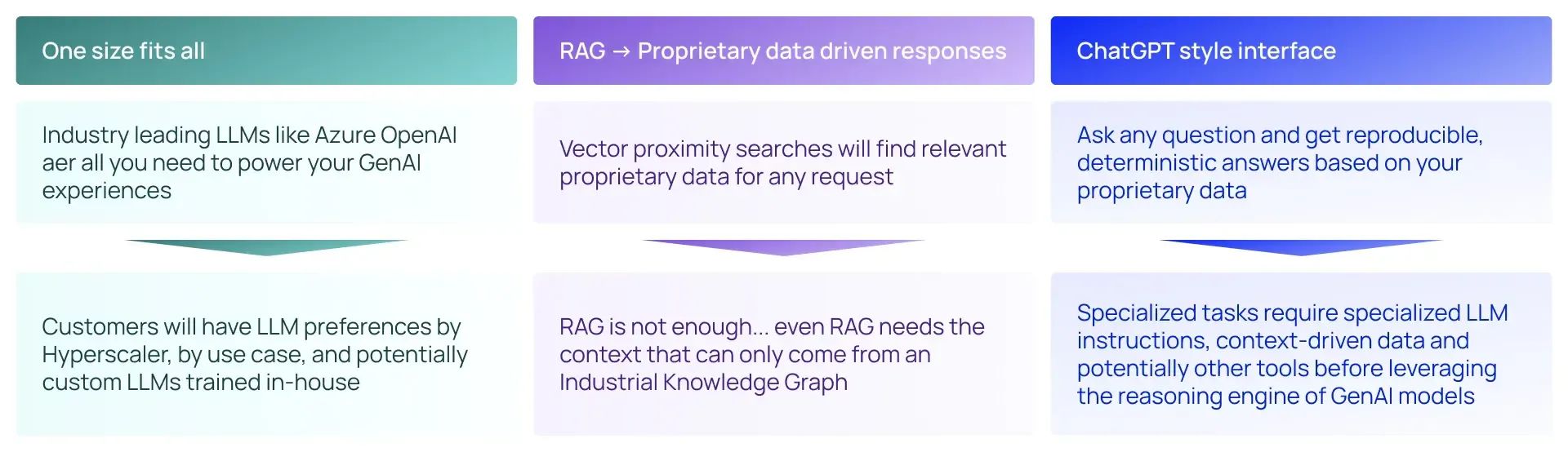
Despite the early learnings, what’s become abundantly clear is that for industrial agents to work and be trusted in industrial domains, they need three things:
- A domain-specific task or objective
- Secure, contextualized data for this objective
- The most appropriate LLM for the task at hand
Until recently, industrial organizations did not prioritize the need for secure, contextualized data foundations, which are critical for training myriad LLMs on relevant data. Today, with support from boards of directors and executives, even legacy industries are investing in teams and technology to bring order and context to their vast amounts of siloed data.
Second, the way users interact with digitally enhanced industrial processes has not been intuitive, making it challenging to actually improve the workflow. In flight, if Iron Man couldn’t speak conversationally with Jarvis and he had to manually look up information with precise terms, his workflow (and mission outcomes) wouldn’t be better. In the field, operator workflows are precise and well-established. Information must be trustworthy and accessible instantly, using handheld devices and simple commands, not lines of SQL code. Technology that doesn’t offer dramatic workflow improvements does not get adopted.
Here’s where things get even more interesting. Gartner predicts: “Large Language Models (LLMs) will become the preferred interface to enterprise data.”2 This means that the effort required for users to access and refine information (once they trust the outcomes) becomes human. So even though an operator may not be able to ask their agents the same breadth of questions as Iron Man could to Jarvis, their interface to answers becomes more human and intuitive than ever before - making it easy to adopt into a workflow.
5 Examples of Industrial Agents
So enough with science fiction. What are some of the types of industrial agents that are already being used now or in the near future?
- Agents for Accelerating Root Cause Analysis: Agents extract information from trend data, alerts, work orders, and more and summarize it automatically in an easy to understand way. The agent provides answers to queries such as, “show me the time series for boiler feed pump A for the last 30 days and highlight what’s out of normal operating parameters.”
- Maintenance Agents for Work Orders: Agents accelerate work order search and interrogation on SAP documents and enables queries such as “Show me all the work orders at facility B which needs to be completed over the next 2 weeks”. One of Cognite’s customers deploys its maintenance agent on more than 250,000 maintenance work orders a year for better maintenance performance.
- Agents for Generating Insights for Drilling & Wells: Agents enable users to perform natural language queries and receive instant analysis on their drilling & wells data. Equinor used an insights agent to help their subject matter experts perform advanced analysis using natural language queries and receive instant data analysis in seconds that would normally have taken hours to compile.
- Agents for integrating simulation workflows: These allow users to run simulations using natural language, e.g., by asking, “What happens with the production if three pumps fail?” and presenting results from the simulator runs in an interpretable manner for business users between simulation runs.
- Agents for parsing documents and populating a data model: Agents extract information from unstructured diagrams and documents and automatically puts them in the right data model with minimal human intervention. AkerBP used a document parser agent like this to save more than 10,000 engineering hours in a year.
Putting these lessons into action
Iron Man didn’t build Jarvis overnight, but we can make several assumptions about what it took to make this high-impact agent. The good news? The journey for industry has many parallels.
- He started with simple access to complex data. Whether you are trying to improve operational dashboards or introduce industrial agents - both start with an industrial data foundation that uses AI to contextualize information at scale. This is the key requirement for any modern industrial digital transformation program. If you’re still struggling in this area, here is a common starting point that has put our customers on fast success tracks.
- He probably used a knowledge graph to contextualize all his data. In the industrial space, LLMs depend on data in context, returning higher accuracy outputs because agents can be trained on narrow data sets based on their explicit objective. Learn more about why this matters.
- He mastered model and agent orchestration: Industrial transformation has a lot of moving pieces, and proper orchestration can make or break a program. While it makes sense to build custom agents in some cases, in others using third-party or Cognite-developed agents could save additional time and money. Regardless of the approach, Cognite can help.
As you can see, there are many considerations for realizing an agent-based industrial future that is reliable, useful, and valuable. Where should you start? With significant hands-on experience providing software to key industries worldwide, Cognite is uniquely positioned to help you start or accelerate your Gen AI journey and get you closer to your own “Jarvis-inspired operations.”
- Science Direct, Industrial Agents, 2015
- Source: Gartner. Quick Answer: Safely Using LLMs With an Active Metadata and Data Fabric Layer. 14 August, 2023
Contact us today or learn more in the resources below.
- Explore Cognite’s newly-launched Atlas AI offering and learn more about AI agents
- Learn what you need to know about Gen AI for industry in our definitive guide
- Discover our Quick Start offering as the ideal starting point and next step